Optimizing data infrastructure for AI era
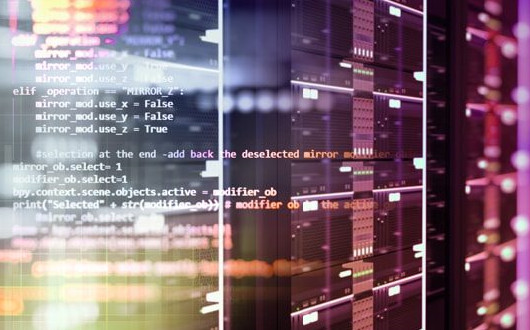
Today, any conversation about artificial intelligence (AI) is bound to include the emergence of ChatGPT, a widely used chatbot built on OpenAI’s GPT series of large language models (LLMs). However, accommodating the demands of this type of generative AI technology in your data center can be challenging.
The chatbot launched late last year and has gained significant attention for its content-generation capabilities. People are utilizing ChatGPT and competing bots from other vendors to obtain answers to complex questions and automate tasks like software code writing and marketing copy production.
Despite the immense potential of this generative AI technology, effectively utilizing foundational models has proven to be difficult. Most existing models have been trained on publicly available data, which makes them less suitable for specific enterprise applications involving sensitive internal documents.
Building LLMs
Enterprises want these models to work with their corporate data, but enterprises can't build them from scratch. The task of building LLMs, such as GPT-3 or GPT-4, requires multiple steps, starting with compute-heavy training that demands hundreds, if not thousands, of expensive GPUs clustered together in data center servers for several weeks or months.
Almost every organization is exploring its own LLM models and use cases. The major providers are already engaged in a race to advance generative AI. But while most leaders consider how to leverage it, they also need to consider how to do so while maintaining control over their most valuable resource - data.
For some organizations, this means bringing the AI computer to the data, not the other way around. To accomplish this, many organizations are looking to build their own models. Providers are racing to build an AI-ready stack and end-to-end toolchain to support generative AI businesses. While cloud solutions are available, the cost of production AI in the cloud can escalate over time. It is vital to consider the TCO of cloud vs. on-prem solutions, and often, high-performance on-prem solutions can provide long-term cost savings while keeping data scientists fully productive.
This is also where an organization’s data infrastructure has to be future-proofed, simple, and scalable enough. The ability to store and protect data while making it agile enough for AI workflows is critical, and not all data storage is up to the task.
In what ways will AI copilots affect data?
First, there’s volume. Generative AI will be one of the most disruptive innovations to affect global data. Conservative estimates predicted 25% compounded data growth year over year from 2022 on—but that was before ChatGPT and image generation exploded. For example, graphic designers can’t physically create 300 unique images in a day, but AI image platforms can. The capabilities of AI are not constrained by physical reality, but the data it produces is. And it needs to live somewhere.
Then, there’s accessibility. According to IDC’s AI Strategies View 2022 study, IT and LOB decision-makers and influencers noted, “Secure availability and accessibility of data is critical to scaling AI initiatives.” Disk can’t keep up. Enterprise all-flash solutions optimized for AI, i.e., have high throughput, parallel and scale-out architecture with data-reducing tech such as compression; offer non-disruptive upgrades, and can scale performance and capacity separately.
AI and ML are the most data-hungry projects in history. Unstructured data, especially photos and video, is notoriously difficult to aggregate and analyze. It requires a platform capable of performing analysis on various data profiles simultaneously or whenever these capabilities are called upon.
And the truth is, while we’d all like to explore more AI projects, we’d also like to reduce footprints in our data centers. Energy to power them isn’t infinite—or cheap. There’s only one way for enterprises to move forward with AI without sacrificing efficiency - flash.
Build a Generative AI-ready Data Center
It is crucial to focus on data infrastructure to build a generative AI-ready data centre. It goes beyond just storing, moving, and manipulating data; the interpretation of data through models and the tooling to build those models becomes a critical component of the software companies' toolkits.
A robust and efficient all-flash storage platform for AI can handle all of the data and tasks generated by NVIDIA GPUs. To maximize the potential of the AI infrastructure, an organization needs a scalable, high-performance, low-latency storage platform that handles lots of data at once (high bandwidth) and can share information amongst many application processes in parallel. This is key to optimizing AI outcomes while minimizing TCO. An AI-ready infrastructure can further accelerate the process by delivering a pre-tested solution, enabling AI and IT teams to focus on innovation rather than deployment.
In conclusion, generative AI for enterprises represents a highly compressed digital transformation initiative. The applicability of LLMs is rapidly evolving, and business and technical leaders need to act swiftly to leverage the latest models customized with internal data while maintaining security. With generative AI, it is evident that data infrastructure needs to be upgraded to meet this transformative technology's demands.
-- Contact us at [email protected]
-
Integration of GIS and BIM can drive development of smart city Dr. Winnie Tang
The China Association for Geospatial Industry and Sciences (“the CAGIS”) released the Top Ten Highlights of China's Geographic Information Industry in 2023, which provides much inspiration. The
-
Equip young people for the future Dr. Winnie Tang
In late February, the inaugural flight of an air taxi from Shenzhen Shekou Cruise Homeport to Zhuhai Jiuzhou Port took only 20 minutes with an estimated one-way ticket price of 200 to 300 yuan per
-
Are we raising a generation of leaders, or of followers? Brian YS Wong
The essence of education is defined not by the facts it imparts, but the potential knowledge it inspires students to individually pursue on their own. Put it this way – the ideal form of education
-
The urgent need for reforms to sex education in Hong Kong Sharon Chau
Nearly one in every four university students (23%) in Hong Kong has been sexually harassed, according to a 2019 report published by the Equal Opportunities Commission (EOC). A 2019 study found that
-
STEAM should be linked to real life Dr. Winnie Tang
In the 2017 Policy Address, STEM (science, technology, engineering and mathematics) education was proposed as one of the eight major directions to promote I&T development. Since then, funding has